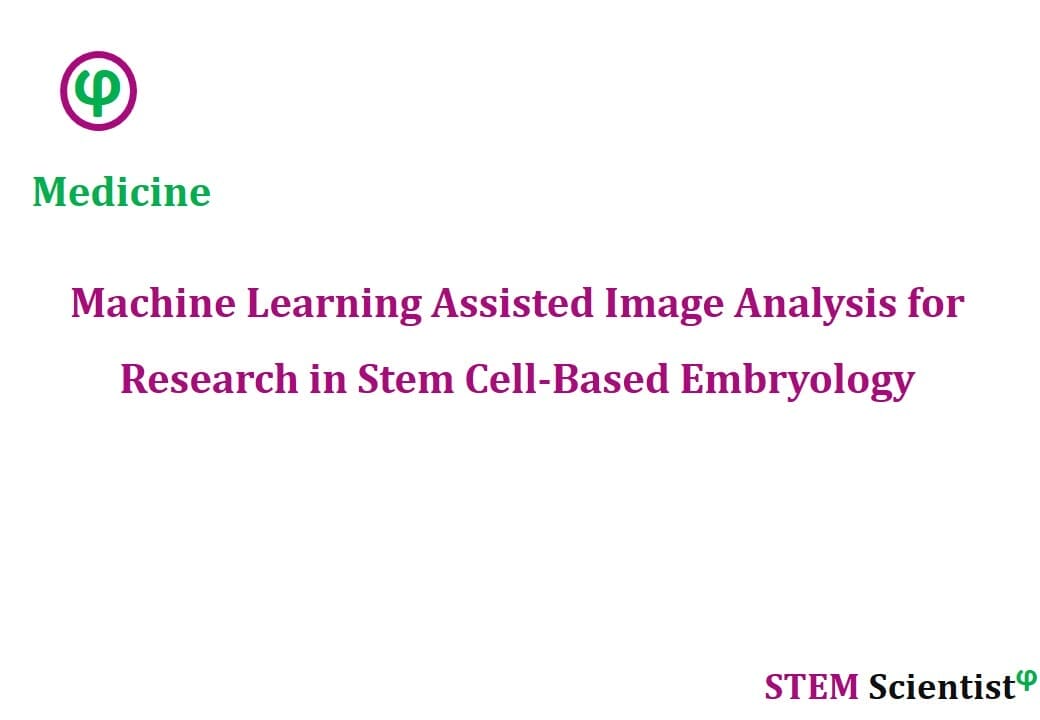
The following study was conducted by Scientists from Center for Stem Cell Biology and Regenerative Medicine, School of Medicine, Tsinghua University, Beijing, China; Tsinghua-Peking Center for Life Sciences, Beijing, China; Mammalian Embryo and Stem Cell Group, Department of Physiology, Development and Neuroscience, University of Cambridge, Downing Street, Cambridge, UK; Department of Genetics, School of Medicine, Yale University, New Haven, CT, USA; Center for Stem Cell Biology and Regenerative Medicine, School of Medicine, Tsinghua University, Beijing, China; Tsinghua-Peking Center for Life Sciences, Beijing, China; Mammalian Embryo and Stem Cell Group, Department of Physiology, Development and Neuroscience, University of Cambridge, Downing Street, Cambridge, UK; California Institute of Technology, Division of Biological Engineering, Pasadena, CA, USA. Study is published in Stem Cell Reports Journal – Cell Press Publishing as detailed below.
Stem Cell Reports Journal – Cell Press Publishing (2021)
Machine Learning-Assisted High-Content Analysis of Pluripotent Stem Cell-Derived Embryos In Vitro
Highlights
- Set up machine learning-assisted image analysis to study stem cell-based embryos
- Mouse iPSCs can form ITS or ITX embryos with TSCs and XEN cells
- Different PSC lines differ in their abilities to form embryo-like structures
- High-content screen identified BMP4 best promoted the morphogenesis of ITS embryos
Summary
Stem cell-based embryo models by cultured pluripotent and extra-embryonic lineage stem cells are novel platforms to model early postimplantation development. We showed that induced pluripotent stem cells (iPSCs) could form ITS (iPSCs and trophectoderm stem cells) and ITX (iPSCs, trophectoderm stem cells, and XEN cells) embryos, resembling the early gastrula embryo developed in vivo. To facilitate the efficient and unbiased analysis of the stem cell-based embryo model, we set up a machine learning workflow to extract multi-dimensional features and perform quantification of ITS embryos using 3D images collected from a high-content screening system. We found that different PSC lines differ in their ability to form embryo-like structures. Through high-content screening of small molecules and cytokines, we identified that BMP4 best promoted the morphogenesis of the ITS embryo. Our study established an innovative strategy to analyze stem cell-based embryo models and uncovered new roles of BMP4 in stem cell-based embryo models.
Source:
Stem Cell Reports Journal – Cell Press Publishing (2021)
URL: https://www.cell.com/stem-cell-reports/fulltext/S2213-6711(21)00148-X
Citation:
Guo, J., Wang, P., Sozen, B., Qiu, H., Zhu, Y., Zhang, X., Ming, J., Zernicka-Goetz, M., Na, J., 2021. Machine learning-assisted high-content analysis of pluripotent stem cell-derived embryos invitro. Stem Cell Reports 16(5), 1331-1346.